A few years ago, I used the data I collected from organizing 2,961 dates (by running speed dating events) to build an AI matchmaking service.
This essay covers the following topics, which can be read in any order:
Part 1: Speed Dating (if limited time, I recommend skipping & starting with part 2)
Economics: Ticket sales, pricing, CAC, revenue, profitability.
Customer Happiness: Structured and free-form feedback from participants.
Marketing: Advertising challenges and the marketing funnel.
Operations: How to run a speed dating event.
Part 2: AI Matchmaker
Data: Personality, IQ, Physical Attractiveness, Identity, etc.
Compatibility Model: Predicting the outcomes of in-person dates.
Product: Designing an AI matchmaking service.
Outcomes & Lessons: Reflections on the project.
Speed Dating: Economics
I ran a total of 30 speed dating events, experimenting with age groups ranging from 22 - 49 years old.
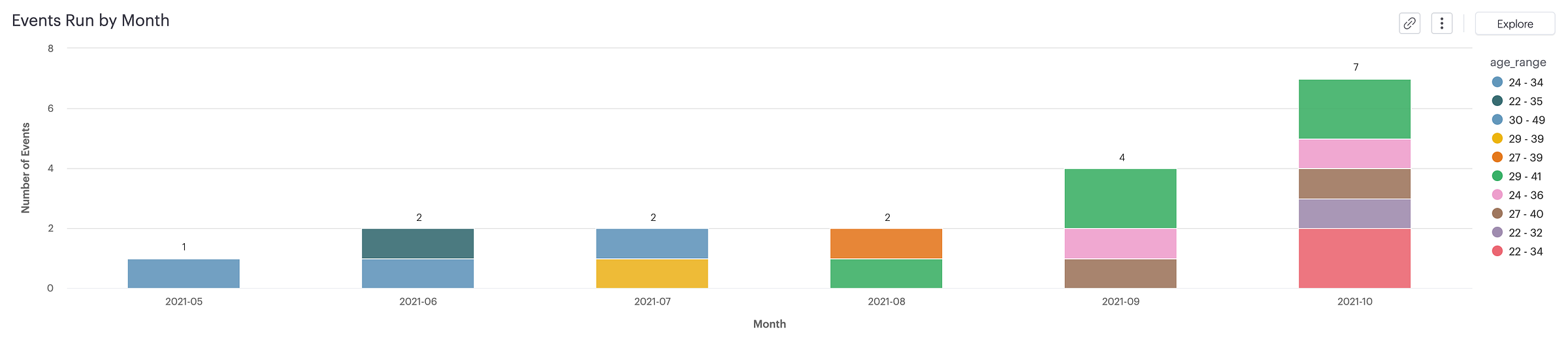
Attendance
My attendance goal for each speed dating event was 10 men and 10 women. I found that these 20-person events were the optimal size. Events significantly larger than that left people feeling overwhelmed, whereas at events much smaller, guests were less likely to find a compatible match, and felt that the event wasn't worth their time.
Maintaining a balanced gender ratio was important. If there was any imbalance, the gender with more attendees had to skip certain rounds. A slight variance of one or two people was generally ok (and could even be appreciated, giving attendees time to use the restroom or grab another drink), but anything beyond that negatively impacted the experience.
Here's what the attendance numbers looked like:
Ticket Prices
Participants were required to purchase tickets before attending an event. I varied ticket prices depending on the demand for seats and the current gender ratio. In some cases, I had to give tickets away for free (e.g. not enough women signing up). In other cases, I sold tickets for as much as $41 (e.g. high demand for men's seats). On average, tickets were $25.
Here are the average ticket prices by gender by month:
CAC
The primary channels for user acquisition were Instagram and Facebook ads. The cost of acquiring a customer (CAC) — i.e. the amount spent on advertising per ticket purchase — varied by age and gender. In general, it was easier to sell tickets to men when running events for younger age groups (but much more difficult to sell to women), and easier to sell tickets to women for older age groups (but more difficult to sell to men).
Here's the average amount I spent on advertising per ticket purchase broken up by gender:
Profitability
On average, speed dating events generated $476 in revenue from ticket sales, incurred $717 in expenses (~90% in advertising, ~10% in sales tax and payment processing fees), resulting in a net loss of $241 per event. 17% of events were profitable.
Speed Dating: Customer Happiness
Event Ratings
I asked people to rate their experience on a 5-star scale after each event. The average rating from 446 reviews was 4.3 out of 5, with men rating the events (4.5 / 5) slightly higher than women (4.1 / 5).
Match Percentage
I also kept track of the percentage of attendees that received at least one mutual match, where both the man and the woman expressed interest in each other after the date. On average, 57% of participants received at least one match during the events.
Mobile App Reviews
The mobile app, available for iOS and Android, had over 3,500 users and an average rating of 4.4 out of 5 during the time that the service was active.
Free-form Feedback
I asked guests to provide free-form feedback as well. Here are some of the positive comments I received:
“Refreshing change of pace from the brainless swiping on mainstream apps. This is like swiping left and right in real life with no pressure.”
“Fun event. Really nice to get out and meet new people. Well organized and the app experience was good.”
“I like that the app has a minimalist feel to promote in-person interactions that shine over all the online dating that's very stale.”
“My event was well planned and really fun! I'll tell my friends about it.”
And here is some negative feedback:
“It was overwhelming to talk continuously.”
“Quality of male candidates needs improvement. Everyone I spoke with said they found it through an ad so maybe refining the ad algorithm to include degrees etc. or even through your app survey so you can separate groups for better matching. Most of the males I spoke with lived with a roommate and had low end jobs. Females don’t want to start a relationship supporting someone (eek!)”
(context: I cover details about the "app survey" in the Data section)
“There were no attractive men there and the only two men I spoke to before leaving were the most socially awkward and it was extremely uncomfortable for me.”
“For $30 I would assume a drink would have been provided.”
Speed Dating: Marketing
Marketing for speed dating events presents a specific challenge: ensuring an equal number of men and women attend a set location at the same time. This is different from selling digital products or services, where location and gender balance aren't usually concerns. It's also distinct from most in-person events (like concerts), which typically focus on local advertising without worrying about their demographic mix.
I ran distinct ad campaigns for men and women for each event. These were geo-targeted to a 30 mile radius around the venue, used custom creative for each gender (e.g. men responded well to simple visuals featuring attractive members of the opposite sex; women did not), and had independent budgets I would tweak depending on ticket availability.
Here's an example of a simple ad that worked well:
After clicking-through on an ad, users were directed to either a landing page or the app store listing, where they would then install the app, create their account, and purchase a ticket.
Here's what the funnel looked like after the first 9 events. The red figures reflect estimated conversion rates at the time, and the green figures show the maximum I was prepared to pay per user to reach a given point in the funnel, assuming I valued a ticket at $20.
I experimented with a variety of channels (including local press, influencers, Snapchat, TikTok, Google Search, Apple App Store), but found that Instagram and Facebook ads were the most consistently effective. A few notes:
TikTok ads were very cost-effective at attracting initial interest, but did not translate into as many conversions further down the funnel relative to other channels. (Possibly because of my limited knowledge: I only briefly played around with TikTok and did not invest significant time into learning the platform & creative.)
Apple Search Ads (ASA) were disappointing (at least when I used them, circa late 2021 - it is possible they have matured since then). The delivery and bidding systems were buggy and there was no transparency or ability to investigate problems. I spoke directly with a few Apple PMs working on ASA, and they seemed as perplexed by their own product as I was.
The ad review system for Instagram and Facebook was frustrating. Ads were often incorrectly flagged for guideline violations, despite using the exact same creative that had passed earlier reviews. The "appeals" process was dysfunctional, and on several occasions, my entire ad account was wrongly suspended. I had to resort to backchanneling with personal contacts on the Facebook team to resolve these lockouts. For small businesses dependent on Meta's advertising for their main source of revenue, this level of unpredictability poses significant risk.
Speed Dating: Operations
Running a speed dating event consisted of the following steps:
Before the Event
Contact a venue, typically a cocktail bar or coffee shop with enough seating. Venues were happy to host for no charge, as events brought them ~20 customers who would typically purchase 2-3 drinks each.
Agree on a date and time.
Launch advertising campaigns about a month in advance.
During the Event
Arrive 30 - 45 minutes early.
Check in with the staff to make sure they have reserved space.
Place table numbers at designated spots so that attendees would know where they should be for each date (guests were assigned to an initial table in the app during their "check in" process).
Greet guests as they arrive. Ease any initial awkwardness while they wait for the event to start.
Kick off the event after everyone grabs their first drink.
Signal to the men to rotate tables every 8-9 minutes by ringing a bell.
Running the events was not rocket science, but did require confidence in wrangling 20 guests, and the ability to handle any issues posed by (occasionally) unruly venues. Dealing directly with owners generally resulted in the best experience, but this was often not possible.
I briefly flirted with having venues run the events themselves so that I did not have to attend each one. While there was some inbound interest, I never felt comfortable relinquishing full control over the experience. Venue staff were often too busy or unreliable to do a great job. In the end, I chose to run all the events myself.
AI Matchmaker: Data
After organizing around 10 events, I lost interest in speed dating for its own sake, given the poor profitability and the operational hassle. However, I realized that the events could produce valuable data as a byproduct. This shifted my focus from speed dating as an end in itself, to a means of training a model for a matchmaking service.
I started to collect two types of data from each event:
the outcome of each date
the attributes and preferences of each participant
Outcomes of In-Person Dates
A single event with 20 attendees yields 100 face-to-face interactions (10 men * 10 women). Each interaction captured the outcome of the date:
did the woman express interest in seeing the man again?
did the man express interest in seeing the woman again?
was there mutual interest?
User Attributes and Preferences
In order to purchase a ticket to an event, users were first required to complete a 100-question compatibility survey in the app. This questionnaire measured attendees':
Big Five Personality: Users were asked to what degree certain phrases described their personality on a scale from "strongly disagree" to "strongly agree". For example:
"warm up quickly to others" (an example of a measure of extraversion)
"hate to seem pushy" (an example of a measure of agreeableness)
Intelligence: I used old SAT questions, which correlate with general measures of intelligence (IQ) somewhere in the range of 0.7 to 0.9. For example: “Each blank in the sentence below indicates a word that has been omitted. Select the option that best completes the sentence: ‘Vernal pools are among the most ------- of ponds: they form as a result of snowmelt and a high water table in winter, and then they ------- by late summer.’”
“transitory . . expand”
“anachronistic . . overflow”
“immutable . . drain”
“itinerant . . teem”
“ephemeral . . evaporate”
Identity: How users identify in key areas of their lives, including political beliefs, religious affiliations, and ethnic backgrounds. It also assessed how much these factors mattered in their romantic and social relationships, for example:
“How important is it that your romantic partner shares your religious beliefs?”
“How comfortable are you being friends with someone that disagrees with you on important political topics?”
Health and Lifestyle: Questions covered alcohol consumption, drug use, exercise habits, etc.
Miscellaneous: Income, height, age, location, how many children they want, whether they already have kids.
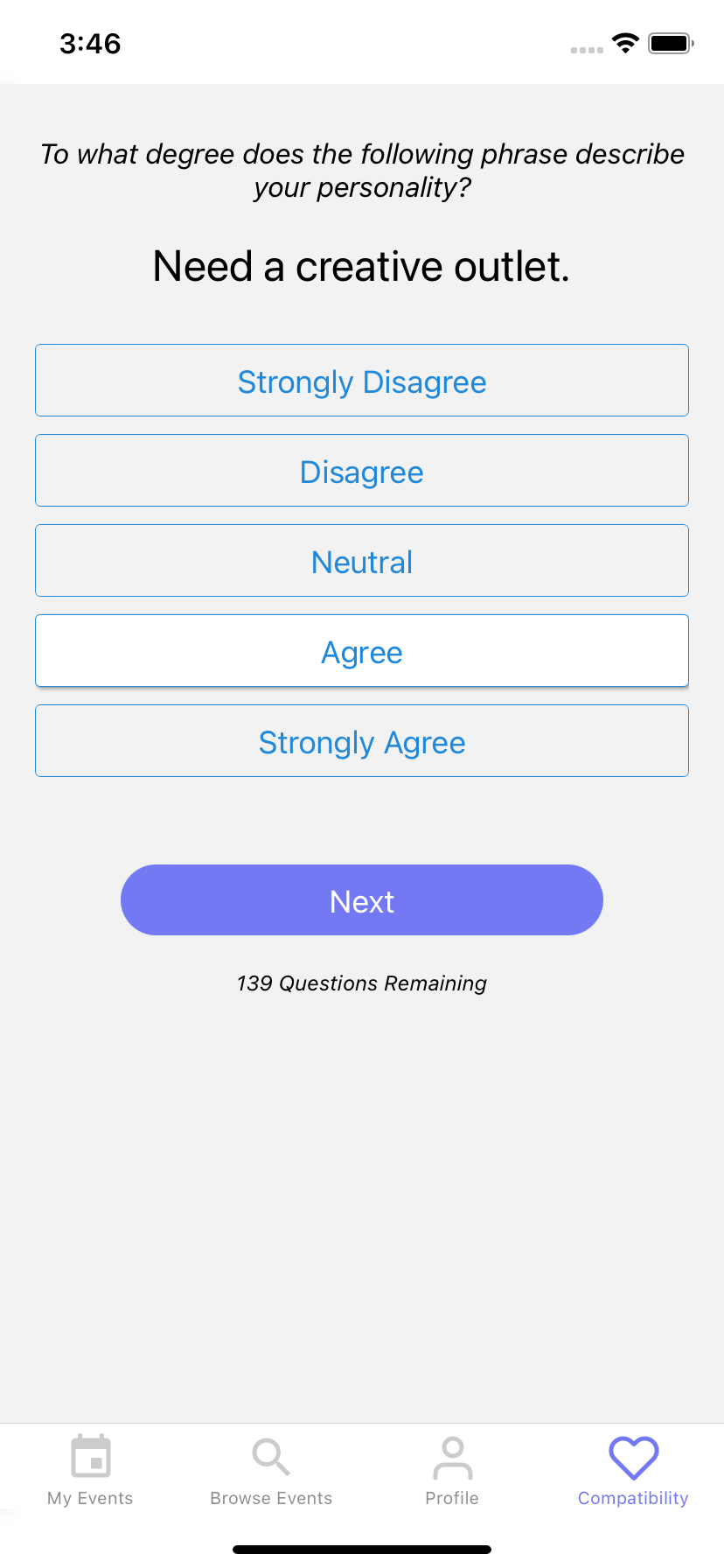
Physical Attractiveness
Physical attractiveness is notably absent from the compatibility survey above. This is one piece of data that dating apps like Tinder acquire easily: their users provide it by swiping on hundreds of other users' pictures. Tinder can then rank profiles by attractiveness based on the percentage of users who swipe right (i.e. express interest) on each.
I was not interested in having my customers mindlessly swipe for 90 minutes per day to collect this data. Instead, I used Prolific, a service that provides rapid collection of human feedback using vetted and customizable demographic groups.
In my case, I recruited heterosexual male participants (of a similar age range as my user base) to rate the physical attractiveness of women, and heterosexual female participants to rate the attractiveness of men.
These recruits from Prolific were directed to a photo rating tool I built. There, they evaluated my users' attractiveness on a scale from 1 to 10, with 1 being the least attractive and 10 the most. Each participant was responsible for rating 60 images, and received compensation upon successful completion of their task.
An average attractiveness score for each user's profile was then calculated (typically using ~11 unique Prolific ratings) from the resulting data.
User Features
Finally, the physical attractiveness data, and the responses to the 100-question compatibility survey were distilled into a user features table, encapsulating a comprehensive set of user attributes and preferences:
I’ve open sourced all my data here.
AI Matchmaker: Compatibility Model
Is it possible to predict meaningful romantic outcomes in advance? Could the emergence of an AI oracle that precisely forecasts the long-term marital success and fulfillment of two complete strangers render modern dating obsolete? Will arranged-marriages-by-AI become the norm?
I began with a more modest topic: Can one accurately predict the outcomes of 8-minute-long first dates?
To answer this question, I trained a compatibility model to predict whether there would be mutual interest after each date given the attributes and preferences of both daters.
In addition to each user's individual characteristics like attractiveness and IQ, I also included features using the principles of assortative mating from evolutionary psychology. To borrow Wikipedia's summary:
Human mating is inherently non-random. Despite the common trope “opposites attract,” humans generally prefer mates who share the same or similar traits.
Birds of a feather flock together: In general, attractive people tend to date other attractive people, smart people tend to date other smart people, and so on.
To capture this idea in the model, I introduced features like physical_attractiveness_rating_diff
to measure the attractiveness gap between the man and the woman, instead of just relying on their individual avg_physical_attractiveness_rating
scores. This approach was extended to other traits, resulting in a set of _diff
features to measure similarity in personality, IQ, values, and lifestyle.
The final training dataset consisted of 1,121 dates with a 12% base rate for mutual interest, and looked something like this:
Model Performance
The random forest model I trained achieved an AUC of 0.7 on the test set. This means that the model had a 70% chance of correctly ranking a randomly chosen successful date higher than an unsuccessful one.
AUC is a bit abstract, so here's another way to think about the model's accuracy: Imagine randomly selecting a man and a woman for a date; the likelihood of mutual interest occurring naturally is about 12%. However, if we use the model to select a pair it ranks in the top 5th percentile of scores, the chance of mutual interest jumps to 45% - nearly a fourfold increase in the probability of a successful date.
The chart below extrapolates this comparison over a series of dates:
Feature Importance
The top ten most important features in the model (out of 118 total) are shown below. Eight of them are _diff
features that measured the similarity (or lack thereof) between the man and the woman:
Improving the Model
Here are some ideas for how the prototype above might be improved:
Quantifying Status: Is it possible to quantify social and professional status? Could PageRank-like features assess the strength and quality of a person's network?
Educational Background: Education-related data was not collected. It would be interesting to pull in things like: level of education, prestige of the institution (e.g. US News Rankings), acceptance rates, average standardized test scores of accepted students, etc.
Intangibles: Is it possible to capture wispy qualities like: communication ability, sense of humor, vibes, the attractiveness of someone's voice? One approach could be asking users to submit short voice recordings in response to prompts like: “Tell me a story from your childhood” or “Tell me about someone that's important to you”. Independent evaluators could then rate these on scales like: “If you were stuck on a road trip with this person for 8 hours, how much do you think you'd enjoy their company?”
Addressing Dishonesty: Self-reported data can be unreliable. What's the best way to handle exaggeration or dishonesty?
Long-Term Tracking: How easy would it be to capture outcomes deeper into the relationship funnel? For example: Is the couple still dating after { 3, 6, 12, ... } months? How happy are they with their relationship? Did they get married?
AI Matchmaker: Product
The Motivation for a New Product
Dating services in 2024 struggle with accuracy, efficiency, and transparency.
Popular dating apps (like Tinder, Hinge, or Bumble) are:
Inaccurate: Matches are based on superficial profiles, leading to a low likelihood of mutual interest after meeting face-to-face.
Inefficient: Misaligned incentives drive these platforms to maximize in-app (vs. real world) outcomes. Users end up spending an hour and a half daily on an activity that rarely results in a date.
Cheap: Apps operate on a freemium model. Most people pay with their time, not their money.
Opaque: It isn't clear why any given user is (or isn't) surfaced in a feed. (e.g. Is just because they paid for it, even if there's no chance of compatibility?)
Human matchmakers are:
Inaccurate: Setting aside instances of outright fraud, matchmakers have difficulty evaluating compatibility because human intuition doesn’t scale. A close mutual friend can play matchmaker, but a stranger can’t.
More Efficient than Apps: Matches are handpicked and dates are coordinated, saving time for the client.
Expensive: Matchmaking packages cost thousands of dollars.
Opaque: Lack of concrete, data-driven justifications for match decisions.
In short, while consumers navigating the dating landscape may opt to trade money for time, they are invariably stuck with inaccurate and opaque services.
I took a stab at building a matchmaker that bridged these gaps. Here's how it worked:
Step One: User Data
The first step involved processing the user's data into a structured set of features:
Step Two: Score all Possible Dates
Next, generate the set of all possible dates (i.e. dates that could happen, but haven't yet), and score each with the compatibility model to obtain the pair's probability of mutual interest.
Step Three: Eligibility
Not all users were eligible for the matchmaking service. If there were not enough "quality" dates for a given user (where I believed that the likelihood of a good outcome warranted investing time and money into meeting), I would place them onto a waitlist. This eligibility process worked as follows:
Filter the user's possible dates using a set of heuristics. For example, many users care about their partner's political views. If either party said it was "very important" that their partner shares their politics, and there was a mismatch, I would exclude those potential dates. Similar approaches were used for location, age, height, religion, and ethnicity.
Filter out potential dates if the compatibility model's probability of mutual interest was too low.
If the remaining number of potential quality dates exceeded a
MINIMUM
threshold, the user was allowed to purchase a matchmaking package. Otherwise, they'd be placed on a waitlist until theMINIMUM
was reached.
Step Four: Organizing Dates
Finally, dates were coordinated for eligible users that purchased a matchmaking package:
AI Matchmaker: Outcomes & Lessons
I rolled out the prototype matchmaking service to a small subset of users in Austin, and ran a trial for a few weeks. Out of 25 eligible users, I had one person purchase a date for $129. But I failed to deliver on their match: I either did not hear back from prospective dates, or was told that they were in a new relationship and no longer interested. I ended up refunding the user for their purchase.
I should have accounted for the fact (obvious, in retrospect) that active user engagement tends to wane over time, even if an account is not explicitly deleted. A profile created six months ago may no longer be relevant, as there is a non-trivial chance that the user has moved on to a relationship.
I wound down the business shortly after this trial. I had reached my threshold for how much of my time and money I was willing to invest.
There's a lot I could have improved: the model's accuracy, the app's design, the level of service, and so on, were far from perfect. But even an omniscient compatibility model is useless if a customer's soulmate isn't an active user on the platform. I failed because of distribution, not because of tech.
This was not a surprise. The fact that distribution is the overwhelming reason for the graveyard of dating startups is not a closely guarded secret. I had known all of this going in. And yet, it was too easy to get distracted. Instead of obsessively focusing on the most critical element for a successful dating service, my attention drifted to evolutionary psychology, papers on human mate choice, collecting and analyzing interesting data, and premature investments in software solutions to problems that could have been manually resolved. While I abstractly understood that these were mistakes at the time, I now grasp their significance in a much more visceral and palpable way.
It is comparatively easy to identify why existing dating services aren't great and how they could be improved. It is much more difficult to figure out how to efficiently acquire a dense, local network of users. Until one has a clear solution to that existential challenge, any investment into a new dating service seems misguided.
This is a problem worth solving: Choosing a spouse is arguably the most important decision one ever makes.
Responses welcome. Email is first name dot last name at gmail.
Do you feel like the speed dating events would've worked better if you guaranteed attendees to be of a certain attractiveness range? I.e. "ages 25-35, guaranteed to be 6+ via photo vetting".
Really awesome work and fascinating article. Reaching out!